Large fortunes have been made and lost with the help of linear regression models ...
In the following we will calculate a linear model on a sample of stock prices and then compare it against stock prices "out of sample".
If you start RStudio, it might show an R script with leftovers from the previous exercise - I suggest you create a new script (menu bar: File > New File > R Script).
We read the R.csv file from step 2 with read.csv:
ryder = read.csv("R.csv",header=T)
and then store the length of the vector ryder$Close:
L = length(ryder$Close).
We calculate two variables we will later use for displays:
maxP = max(ryder$Close) + 5.0
minP = min(ryder$Close) - 5.0
The file should contain more than 250 data points (one year of stock prices) and we use the first 200 days for in sample estimation.
days = 1:200
prc = ryder$Close[days]
Notice that ryder$Close is a vector and ryder$Close[1] is the first element we want and ryder$Close[200] the last of the in-sample period. ryder$Close[days] is equivalent to ryder$Close[1:200] and therefore prc is a vector which stores the first 200 elements of ryder$Close.
This slicing of vectors is quite often used and would work even if the elements of days would not be sequentially ordered.
Now we can plot the prices as a function of each day:
plot( days, prc, ylim = c(minP,maxP) )
We calculate the linear regression model with the lm() procedure:
mdl = lm( prc ~ days)
Notice the ~ used in the procedure call (which may or may not not be easy to find on a non-US keyboard).
We can print a summary of the linear model with
summary(mdl)
and we get the two coefficients (intercept and slope) using coef(mdl), which returns a vector.
Therefore we can calculate the linear model as
lin = coef(mdl)[1] + coef(mdl)[2]*days
and add the line to our plot with the lines() procedure:
lines( days, lin, type="l", col="red")
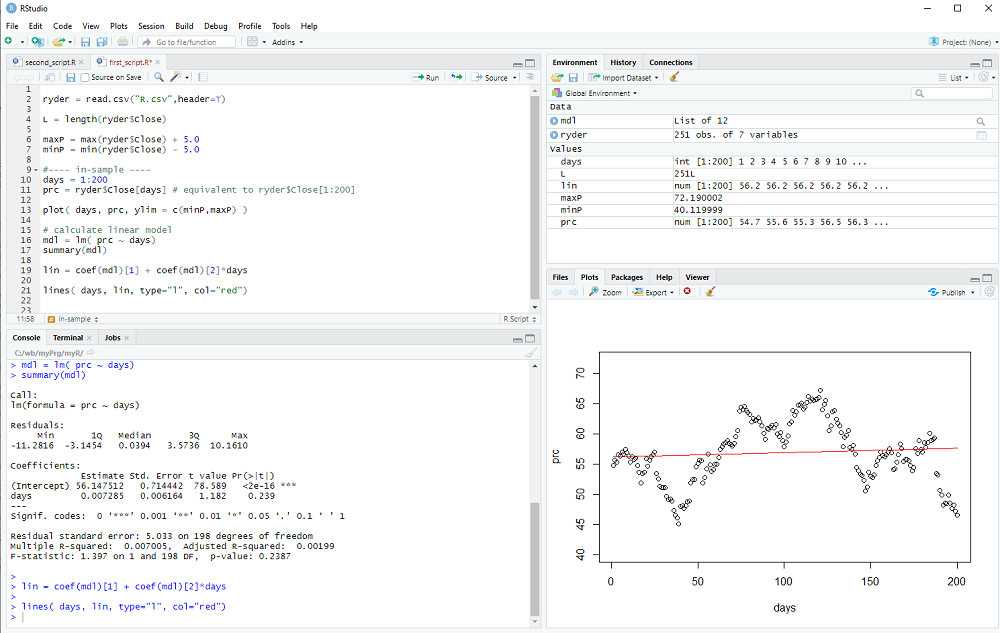
You can either execute your script step by step with Ctrl-Enter or type the script first and then execute the whole thing by selecting from the menu: Code > Run Region > Run All
Now we look at the out-of-sample data:
days = 201:L
prc = ryder$Close[days]
and display it with
plot( days, prc, ylim = c(minP,maxP) )
We calculate the out-of-sample model
lin = coef(mdl)[1] + coef(mdl)[2]*days
and add the line to the plot
lines( days, lin, type="l", col="red")
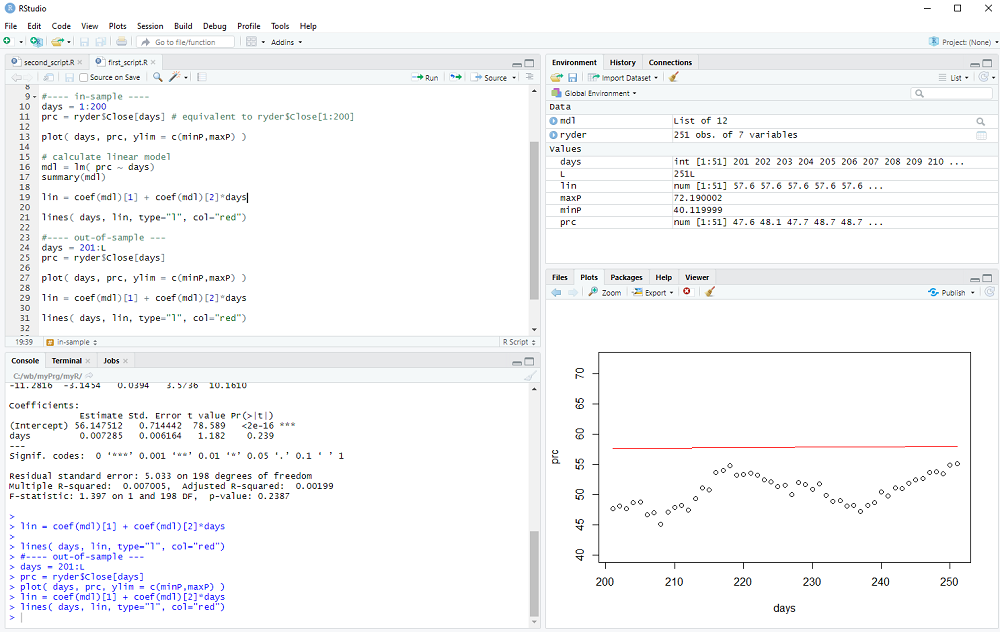
We could now calculate the out-of-sample error (it is quite obvious that the error exceeds the variance of the data in this example 8-) and perhaps repeat the procedure for many different stocks and time periods to check if the linear model has any useful predictive value.
However, this concludes step 4 of my introduction
exercise: Repeat this regression exercise, but use the Volume instead of the Close ...